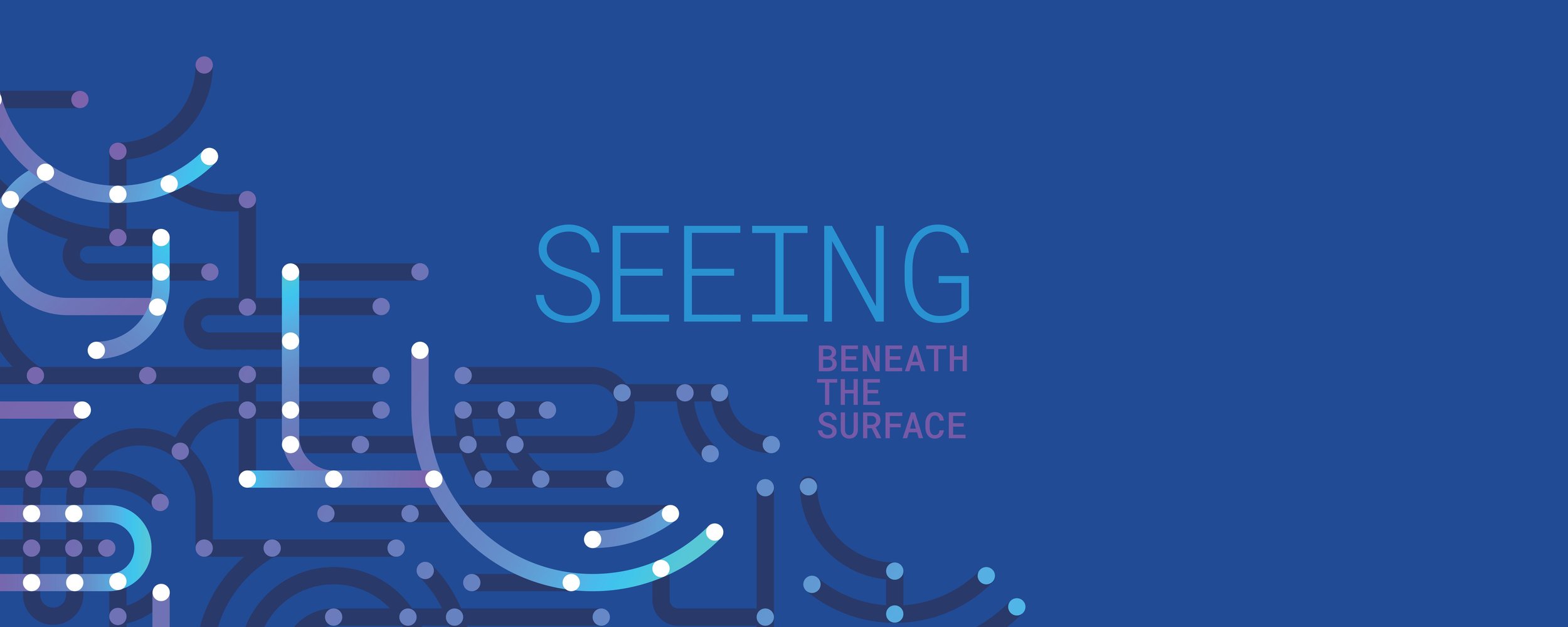
SEEING BENEATH THE SURFACE
Convolutional Neural Network Framework Examines Ultrasound Images and Improves Detection of Subcutaneous Cancer Cells
MEGAN HARRIS
Precision is paramount in most stages of scientific research. A Robotics Institute’s weekly roundtable discussion recently honed in on an essential idea: what exactly does it mean for an image to be fuzzy?
At its core, an ultrasound is an image of gathered high-frequency sound waves. Each component part is a collection of pixels, and until now, humans have been tasked with interpreting them to make or better understand a medical diagnosis.
But what if AI could do better.
“Ultrasound is the safest, cheapest and fastest medical imaging modality, but it’s also arguably the worst in terms of image clarity,” said John Galeotti, a systems scientist with CMU’s Robotics Institute and adjunct assistant professor in biomedical engineering. “The research here is ultimately about teaching AI to process detail in an ultrasound that typically doesn’t get used. So just by having that extra information, the AI can make better conclusions.”
Through interdisciplinary research, collaborations and teaching, Galeotti has been working for years to improve patient outcomes by focusing on the tools of science and medicine. His most recent work introduces W-Net, a novel Convolutional Neural Network (CNN) framework that uses raw ultrasound waveforms in addition to the black-and-white ultrasound image to semantically segment and label tissues for anatomical, pathological or other diagnostic purposes. Initial findings were published in the journal Medical Image Analysis in February of 2022.
“To our knowledge, no one has ever seriously tried to ascribe meaning to every pixel of an ultrasound on a regular, ongoing basis, so it’s not that we crossed some invisible barrier of never being able to do this and now we can,“ said Galeotti. ”It's more that AI was pretty bad at this, and thanks to W-Net, it has the potential to be much better.”
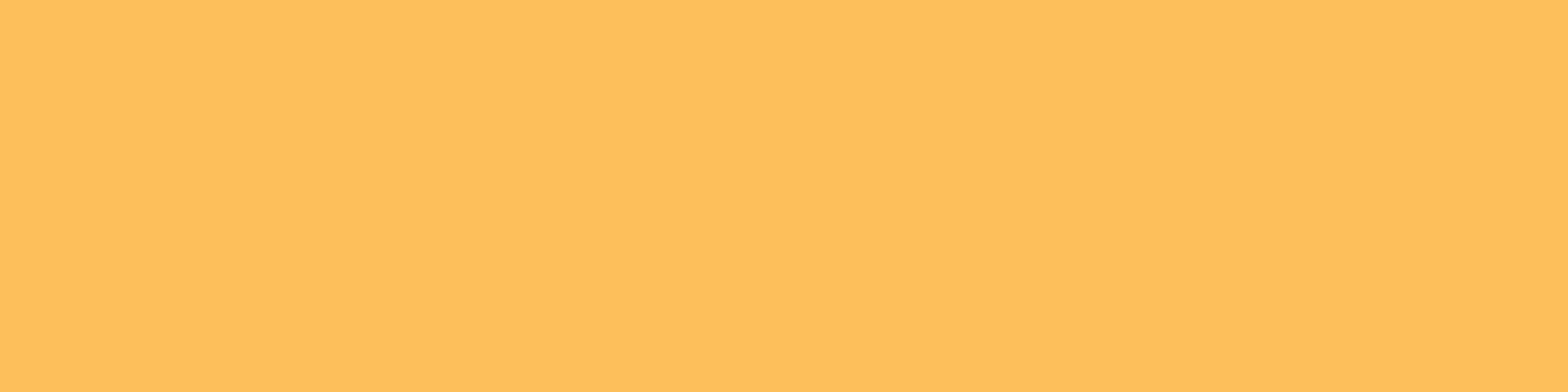
The research here is ultimately about teaching AI to process detail in an ultrasound that typically doesn’t get used.
For even highly trained humans, healthy or distressed tissue can be tough to confidently discern. Fuzziness in the image could indicate disease or pathology, but how much fuzziness is worrisome? Beyond the subcutaneous layer, brightness, breaks and indents in various lines may suggest a need for further testing.
Either way, the technology is limited and patient care has been dictated by subjective human assessment.
W-Net goes further by attempting to label every pixel without the use of a predetermined background classification for the entire static image. Galeotti’s team recently applied the idea to breast tumor detection, which in tests, outperformed established diagnostic frameworks.
The group has since returned to pulmonary applications, which is where much of their work has focused since the pandemic took hold in 2020.It all started with Baltimore-based plastic surgeon Dr. Ricardo Rodriguez, who was looking for a better way to monitor the treatment of irradiated breast tissue after the injection of reconstructive fat cells. He wanted to see how the fat changed or helped heal the damaged tissue, so he called and wrote letters to imaging specialists near and far.
Galeotti answered that call.
“As a clinician, I used to look at an ultrasound and it was completely unintelligible. Like a snowstorm,” he said. “I see something; it’s obviously there. But our brains aren’t equipped to process it.” Rodriguez wondered, what if a screen could better show people what they’re looking at?
“We needed to create a deep-learning model that could understand the reading and present it to both clinicians and patients on a screen. And not in a way that requires years of training. Let’s make it obvious so you can interact with it, emotionally and intellectually.”
Gautam Gare, a Ph.D. candidate still working alongside Galeotti got an early crash course in radiography — learning from medical professionals how to label bits of lung scans and recognize markers for disease or pathology. He’s 0processed thousands of individual scans, each bringing the AI system closer to performing the same tasks on its own.
“It was a big learning curve,” Gare said. “When I started, I didn't even know what an ultrasound image looked like. Now I understand it better, and the potential for this research is still really exciting. No one is exploring the raw data exactly the way we are.”
In recent months, much of that labeling work has moved to a team of pulmonary specialists at Louisiana State University in Baton Rouge under the care of professor of medicine and physiology Dr. Ben deBoisblanc. Finding a pulmonary partner took time, Rodriguez said, until he turned to his own family.
In addition to serving as the director of clinical care services at the Medical Center of Louisiana, deBoisblanc is also Rodriguez’s wife’s cousin. It was a happy accident when the two crossed academic paths; deBoisblanc said he wasn’t initially interested in ultrasound, but as he learned more about how technology could be applied — and got to know the team — he went all-in.
“I’ve always been a geek — to this day, I’ll sit on my spin bike and read journal abstracts for fun — but this team is different. We have so much respect for each other, and a deep sense of collaboration and curiosity. They’re easy to work with, and they’re a lot of fun.”
With deBoisblanc’s group of about a dozen LSU clinicians sharing and labeling lung scans, Galeotti’s team has been able to refine the process. On Thursdays, the primary team of Galeotti, Gare, Rodriguez and deBoisblanc can virtually review the scans for biomarkers, or labels, often with the help of the clinicians themselves. With more scans come adaptations in the definitions. These meet-ups give them a chance to adjust, and in turn, improve the technology.
Though it remains labor intensive, Rodriguez said it’s all a part of step one — teaching the AI to understand the signal. Step two involves translating that data into an image physicians and patients recognize as a better version of an ultrasound. deBoisblanc takes it a step further.
“As the technology improves, we’ll need to look for clinical correlations, and that’s where we are now. The third part is testing those outputs. We’re not even close to that yet.” But to his mind, the market is ready for their work.
“Twenty years ago, you had to be a radiologist to understand this stuff. Now I’m pulling a little unit around from patient to patient on my morning rounds. Imagine a battlefield or an ambulance en route to a hospital, where someone with little to no training could point a device at an injured or incapacitated person and know immediately what might be wrong. It’s the perfect time to let AI assist from here.”